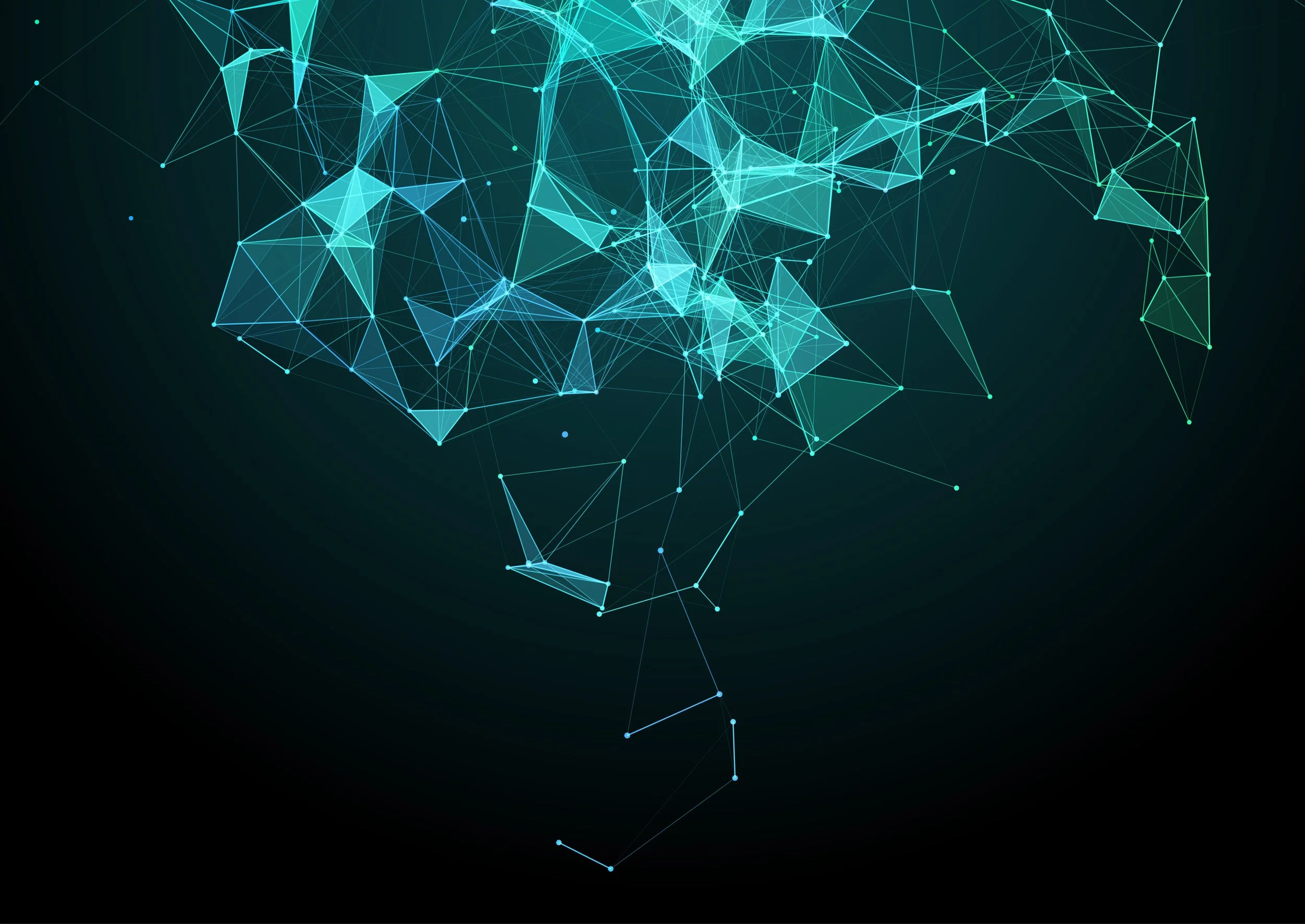
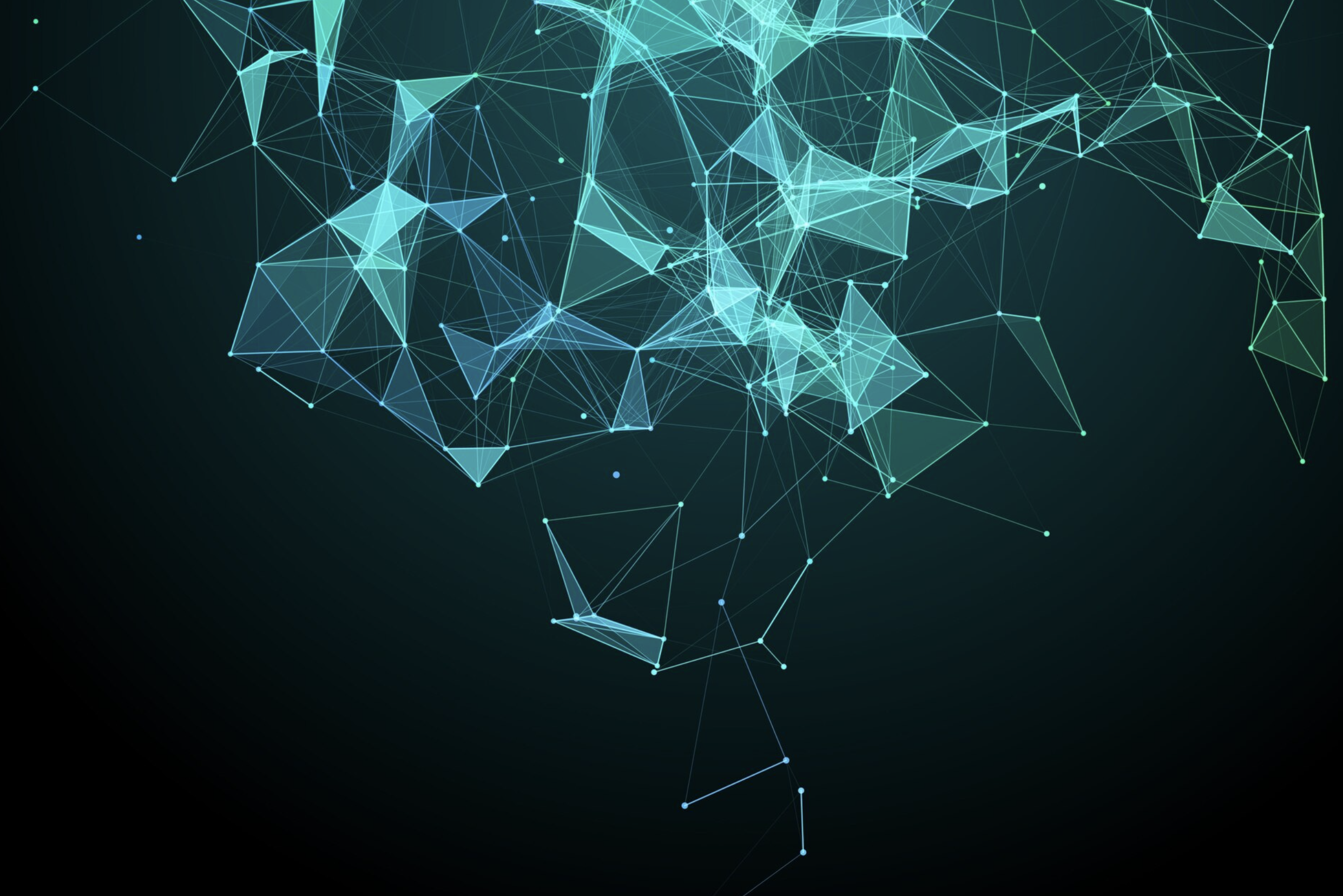
What do we offer?
Hit identification
We scan over 10 billion ready-to-order molecules in a single day.
Unlike traditional structure-based screening, our AI-driven approach uses protein sequences alone, dramatically increasing throughput and scalability.
Our ultra-fast virtual screening dramatically cuts down the time and cost of your drug discovery pipeline.
Want to explore off-target effects and toxicity? With our tech, it has never been easier to validate top-ranked molecules across other proteins.
Hit refinement
Once hits are identified, we help you turn them into optimized leads.
Validate leads rapidly by iterating through make/test/refine cycles, accelerating your R&D timelines, reducing costs, and avoiding surprises.
Go from AI-prioritized hits to biologically validated leads, cutting months off your timelines.
Proprietary data integration
Of course, your proprietary data remains completely secure and exclusively yours.
We seamlessly integrate your private database into our pipeline to achieve personalised predictions. These are precisely tailored to your unique therapeutic objectives, while fully respecting your privacy.
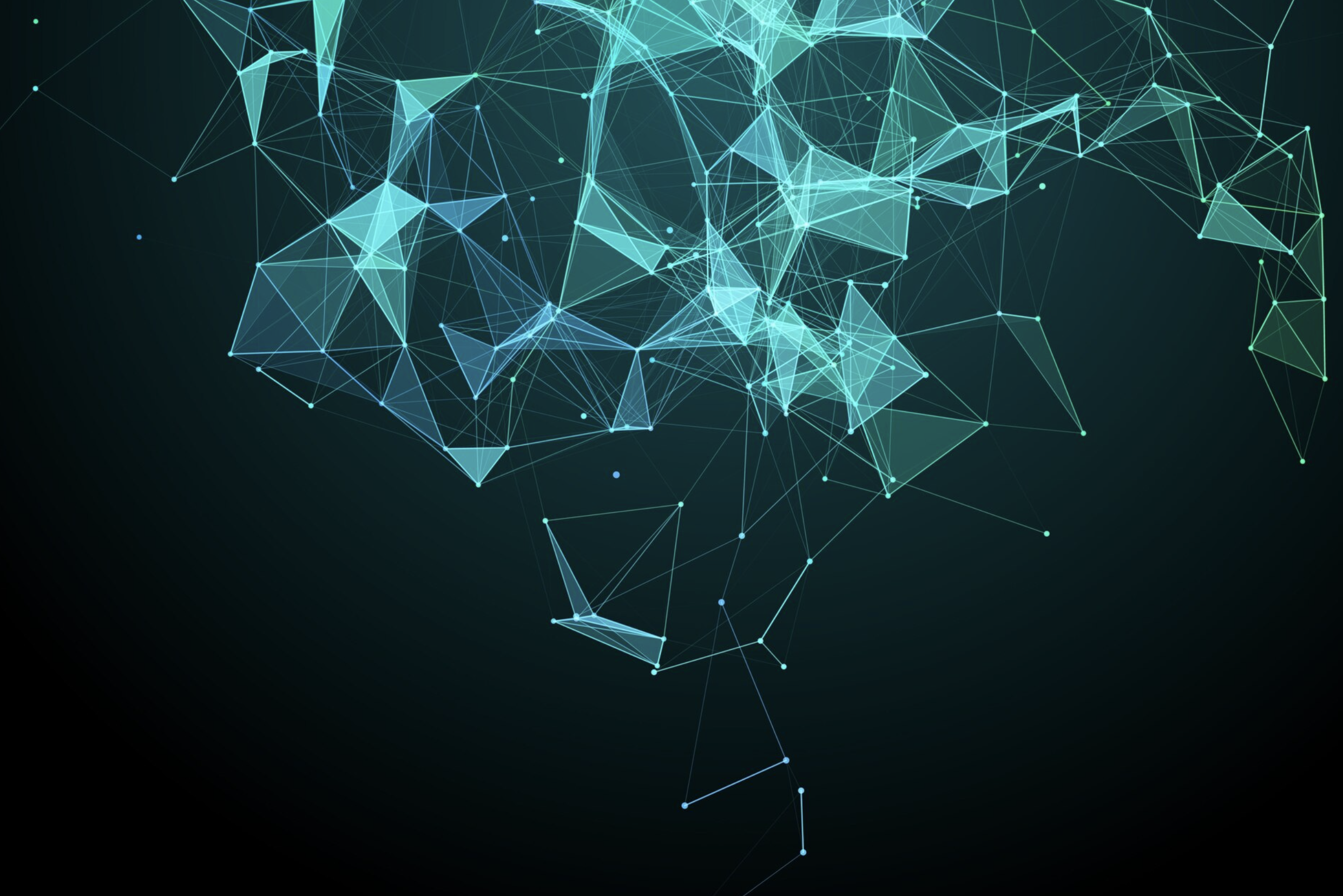
What would you get?
USPs
Thanks to our technology, you only need a simple protein sequence to access novel chemistry and previously unreachable targets.
You can explore over 10 billion molecules in a single day to accelerate drug discovery with greater precision, lower costs, and reduced risk.
But more specifically
A ready-to-order SMILES list containing the predicted hits
(customer defined size)
With model scores, prediction confidence,
and refinement statistics
Enhance your insights with optional add-ons:
Property-based filtering
Selectivity and off-target insights
Toxicity risk assessment
Predicted binding residues
Scaffold diversity analysis
Novelty scoring
Structure-based rescoring
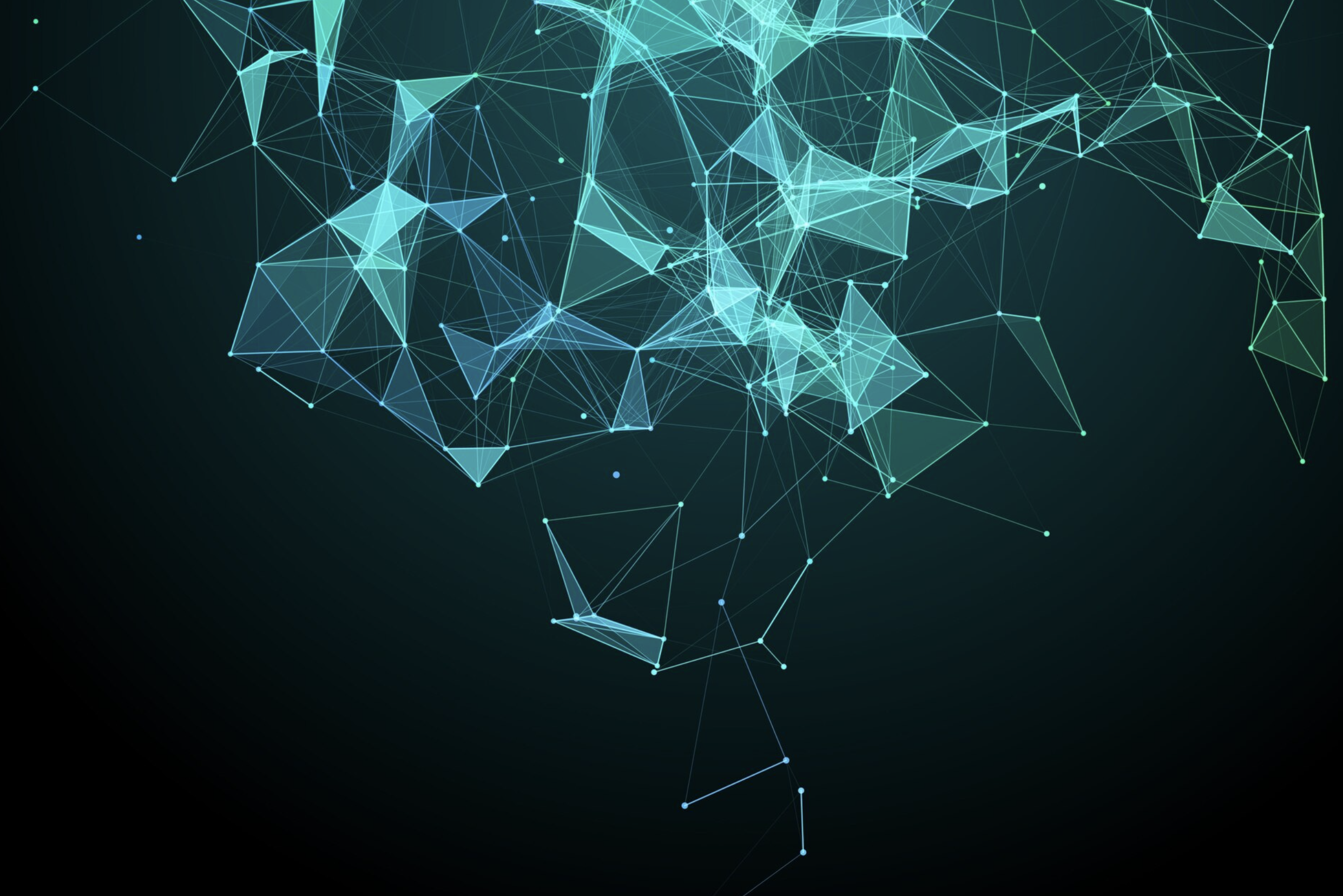
How do we do it?
The science behind it
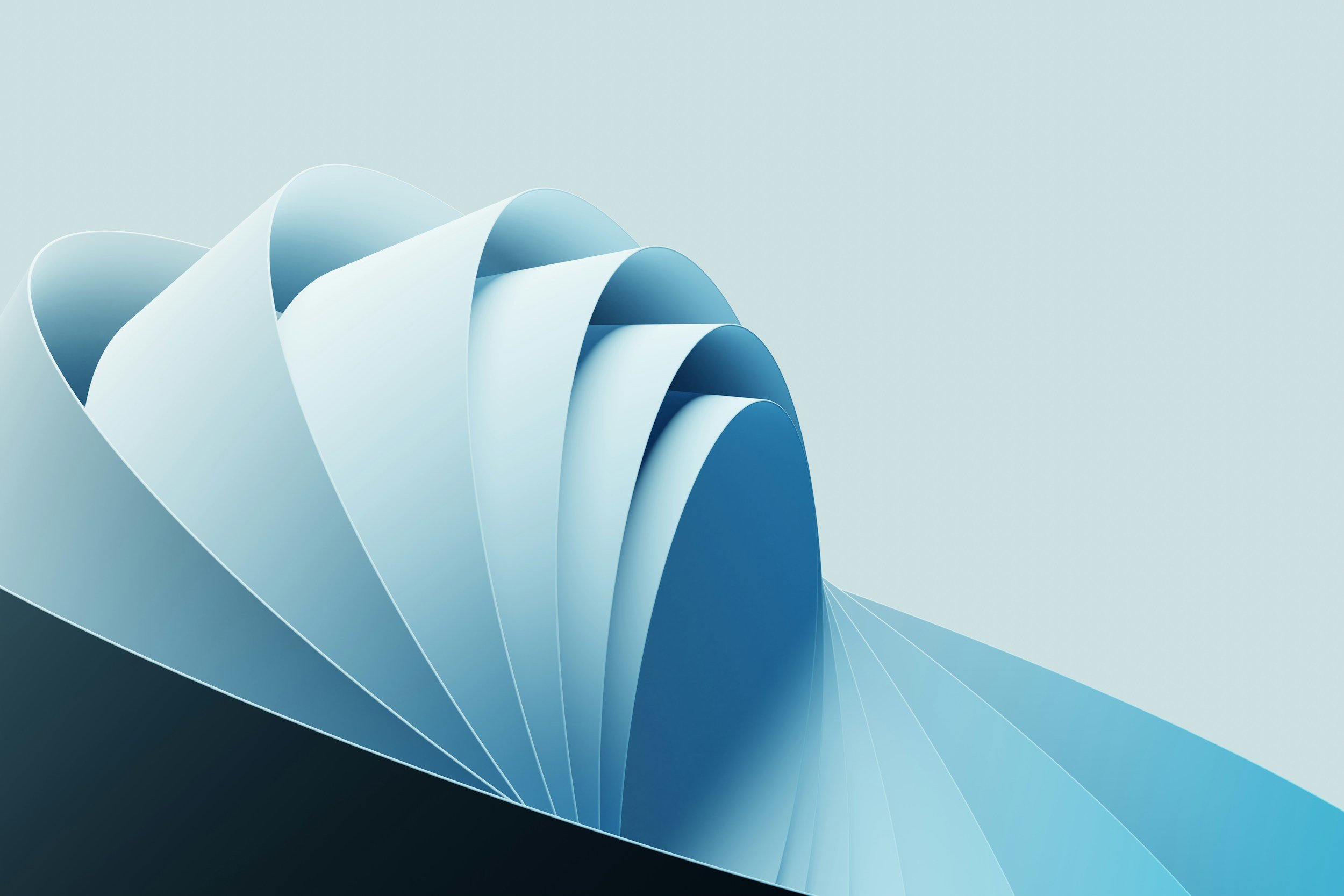
Our AI model is designed to predict which molecules are likely to bind to which proteins, without needing 3D structures.
Instead of complex structural data, it uses simple text formats: SMILES for molecules and amino acid sequences for proteins.
CC1CCCC2(C1(CCCC2)O)C
MEIVSTGNETITEFVLLGFYDIPELHFLFFIVFTAVYVFIIIGNMLIIVAVVSSQRLHKPMYIFLANLSFLDILYTSAVMPKMLEGFLQEATISVAGCLLQFFIFGSLATAECLLLAVMAYDRYLAICYPLHYPLLMGPRRYMGLVVTTWLSGFVVDGLVVALVAQLRFCGPNHIDQFYCDFMLFVGLACSDPRVAQVTTLILSVFCLTIPFGLILTSYARIVVAVLRVPAGASRRRAFSTCSSHLAVVTTFYGTLMIFYVAPSAVHSQLLSKVFSLLYTVVTPLFNPVIYTMRNKEVHQALRKILCIKQTETLD
The model uses a self-supervised learning approach to understand protein-ligand activity.
This means it learns by comparing many examples of binding and non-binding pairs using a method called contrastive learning. It then builds a shared space, like a map, where proteins and molecules that are likely to bind end up close together.
We have used over 80 million protein–ligand activity data points from public databases like PubChem, ChEMBL, and BindingDB to teach the model this “binding logic”.
To ensure quality, the data has been carefully selected, prepared, and exhaustively curated by experts, resulting in a gold-standard dataset.
The result?
We can screen billions of compounds in under a day, identifying the most promising ones for a given protein, bypass IP restrictions, and unlock targets that are inaccessible to structure-based methods.
But... How can sequences alone predict binding?
Protein sequences carry hidden predictive signals about binding behavior, signals that traditional structure-based methods miss.
Our AI captures these patterns through massive-scale learning, giving you actionable insights even when structures are unavailable.In short: Sequences → Patterns → Binding predictions → Hits
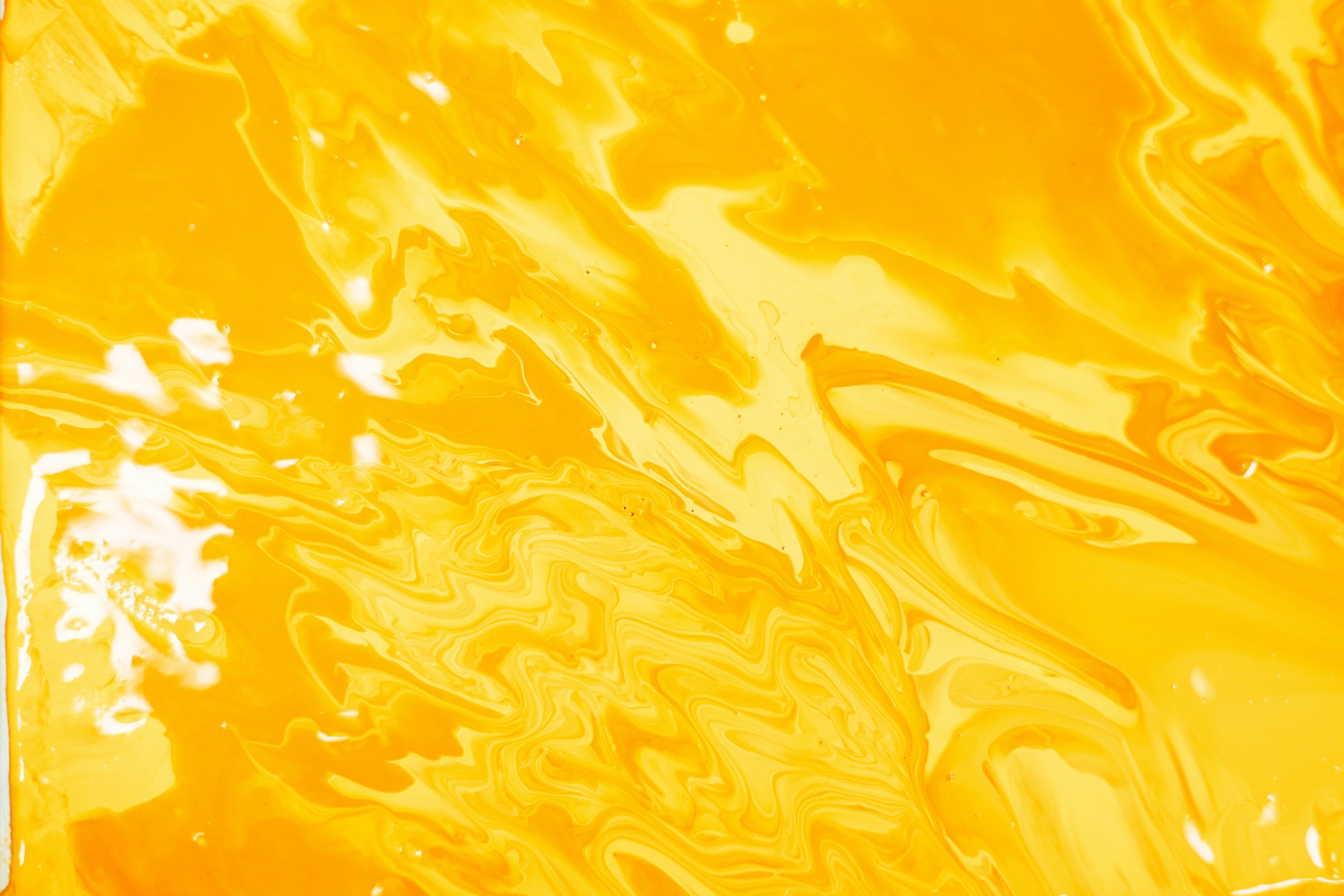
All this is, of course, just a simplified glimpse of what we do. If you’re curious to dive deeper or have any questions, we’d love to hear from you. Don’t hesitate to reach out!